The Power of Machine Learning Labeling Services
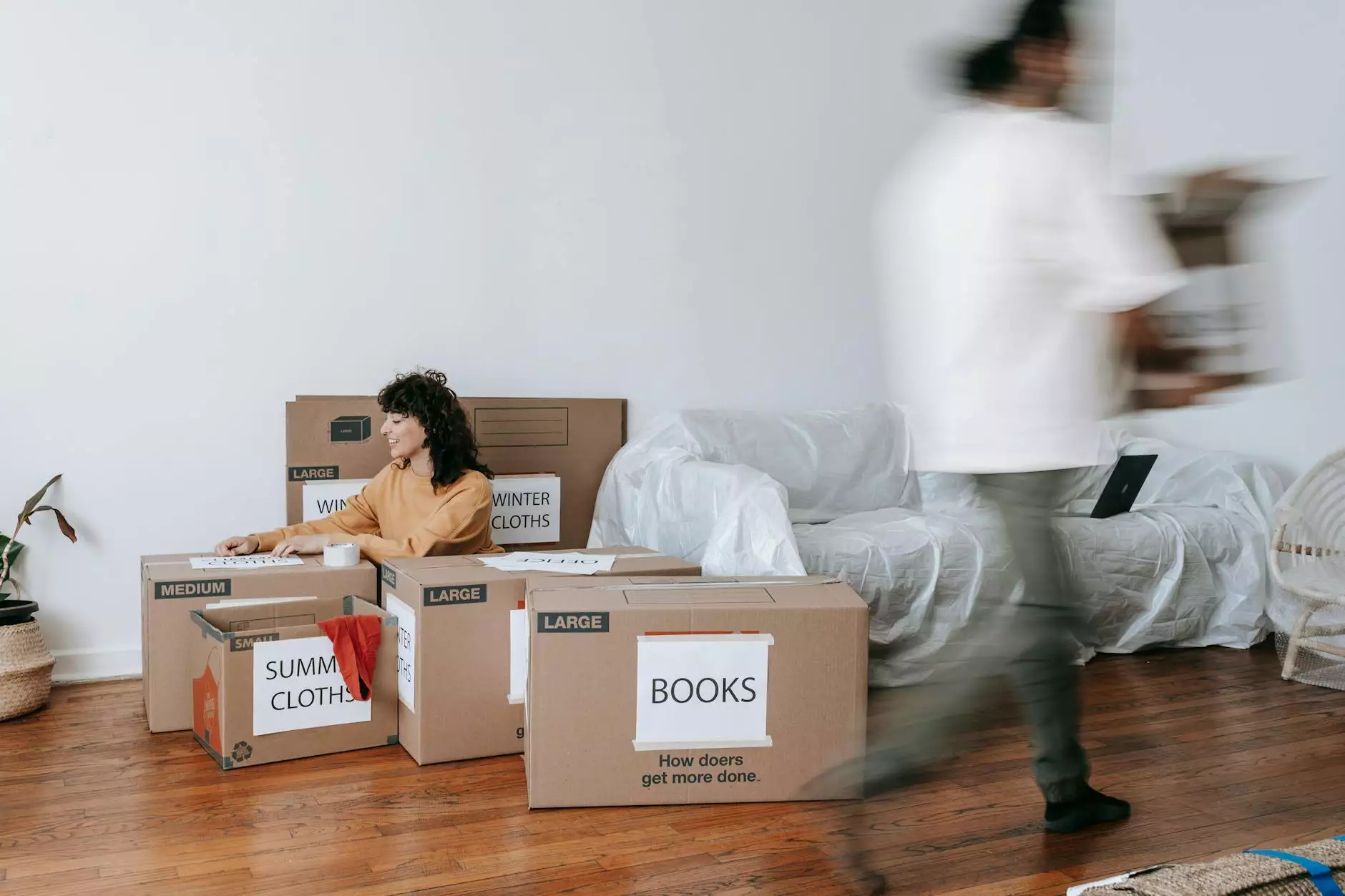
Machine learning labeling services are a critical component in the landscape of artificial intelligence and data processing. As businesses across various sectors strive to harness the capabilities of machine learning, the demand for precise and efficient data annotation continues to surge. This article explores the essentials of data annotation, the unparalleled advantages of leveraging machine learning labeling services, and how platforms like KeyLabs.ai are setting new standards in the industry.
Understanding Data Annotation
Data annotation is the process of labeling data to make it easily analyzable and usable for machine learning algorithms. This foundational step is pivotal in training AI models to perform tasks such as image recognition, natural language processing, and predictive analytics. The accuracy and quality of this labeling directly influence the performance and reliability of AI systems.
The Role of Data Annotation Tools and Platforms
With the increasing complexity of data, companies are turning to advanced data annotation tools and data annotation platforms to facilitate the labeling process. KeyLabs.ai offers state-of-the-art solutions that enhance the efficiency and accuracy of data annotation operations. Let's delve into the key features that make such platforms indispensable:
- User-Friendly Interface: A streamlined interface simplifies the labeling process, allowing annotators to work efficiently without extensive training.
- Scalability: Machine learning labeling services can easily scale to accommodate large datasets, ensuring quick turnaround times.
- Quality Control: Implementing multiple layers of verification ensures that the labeled data maintains high-quality standards.
- Customizability: Tailoring the annotation process to meet specific project needs enhances relevance and precision.
- Integration: Seamless integration with existing workflows and tools streamlines operations and boosts productivity.
Benefits of Machine Learning Labeling Services
Investing in machine learning labeling services offers numerous advantages that can propel your business ahead of the competition. Here are some compelling benefits:
1. Enhanced Data Quality
The efficacy of any machine learning model hinges on the quality of the training data. Well-labeled datasets allow models to learn accurately, reducing the likelihood of errors and improving overall performance.
2. Time and Cost Efficiency
Outsourcing your data annotation needs to specialized services like those offered by KeyLabs.ai can save considerable time and resources. Automation and sophisticated tools expedite the labeling process, allowing your team to focus on core business activities.
3. Access to Expertise
Partnering with a reputable machine learning labeling service means tapping into expert knowledge to enhance the quality of your data. This expertise ensures that even the most complex data types are annotated correctly.
4. Accelerated Product Development
With timely access to labeled data, businesses can accelerate the development of AI-driven products and services. Speed to market is often a decisive factor in gaining competitive advantage.
5. Improved Model Performance
A well-trained model leads to better predictions and insights, which can drive strategic decisions. The relationship between quality labeled data and superior model performance cannot be overstated.
Key Considerations When Choosing a Machine Learning Labeling Service
When selecting a provider for machine learning labeling services, it's essential to weigh several factors to ensure a suitable match for your business needs:
1. Expertise and Experience
Evaluate the provider's track record in delivering high-quality data annotation across various industries. Their experience can be a significant predictor of success in meeting your specific requirements.
2. Technology and Tools
Examine the technology used by the annotation service. Advanced tools equipped with AI and machine learning capabilities can greatly enhance the labeling process.
3. Quality Assurance Processes
Inquire about the quality control measures implemented by the provider. A reputable service will have stringent processes to ensure high precision and reduced error rates in the labeled data.
4. Flexibility and Customization
Your project may have unique needs concerning labeling formats, data types, or turnaround times. Ensure the service can customize its approach to meet your objectives.
5. Pricing Structure
Understand the pricing model—whether it's per label, per project, or subscription-based. The goal is to find a balance between affordability and quality.
Examples of Effective Data Annotation Use Cases
To illustrate the transformative power of machine learning labeling services, here are several use cases across various domains:
1. Healthcare
In the healthcare sector, precise data annotation is vital for developing diagnostic tools and patient management systems. For example, machine learning models trained on annotated medical images can identify diseases with unprecedented accuracy.
2. Autonomous Vehicles
Self-driving cars rely heavily on labeled data to interpret their surroundings. From image recognition of pedestrians to identifying road signs, accurate data annotation is crucial for improving safety and performance in autonomous driving systems.
3. E-commerce
In e-commerce, machine learning labeling services can enhance product search and recommendation systems. Accurately labeled product images and descriptions lead to better matching algorithms and improved customer experiences.
4. Social Media and Content Moderation
Platforms use data annotation to enhance content moderation systems. By labeling types of content and user interactions, social media platforms ensure compliance with legal standards and improve user satisfaction.
Implementing an Effective Data Annotation Strategy
To maximize the benefits of machine learning labeling services, implementing a strategic approach is essential. Here are some steps to create an effective data annotation strategy:
1. Define Objectives
Clearly articulate the goals you wish to achieve with your labeling project. Having defined objectives helps guide the annotation process and ensures alignment with your overall business strategy.
2. Select the Right Partner
Choose your data annotation service provider carefully. Consider the factors discussed earlier to ensure you select a partner capable of meeting your needs effectively.
3. Design a Comprehensive Data Plan
Develop a plan detailing the data to be annotated, the labeling requirements, and the expected timeline. A well-structured plan facilitates smooth operations and minimizes delays.
4. Train Annotators
Providing comprehensive training to annotators enhances data quality. Ensure they understand the nuances of the labeling tasks and the importance of accuracy in the process.
5. Monitor and Review
Regularly evaluating the quality of the annotations ensures standards are met. Establish feedback loops to address issues promptly and refine the process over time.
The Future of Machine Learning Labeling Services
As machine learning and artificial intelligence continue to evolve, so too will the methods and technologies used in data annotation. Organizations will increasingly adopt AI-driven annotation tools that can automate routine tasks while maintaining high-quality standards. At KeyLabs.ai, we are at the forefront of these developments, continuously innovating to provide the best machine learning labeling services.
Conclusion
In summary, machine learning labeling services are indispensable for organizations looking to leverage AI's full potential. With high-quality labeled data, businesses can greatly increase the effectiveness of their machine learning models, ensure rapid product development, and drive innovation across sectors. By partnering with experts like KeyLabs.ai, companies can achieve their AI objectives while reaping the benefits of efficient and accurate data annotation. Embrace the future of data annotation and unlock the true power of your data today.